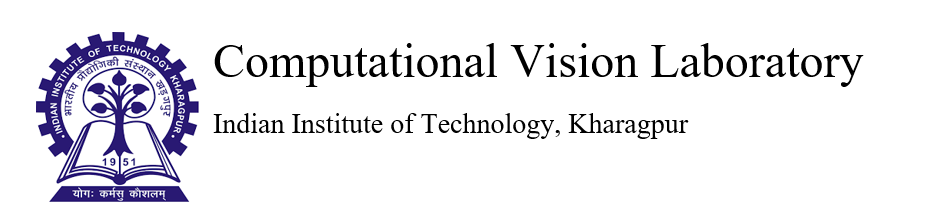
Nrityabodha (Understanding dance)
Indian classical dance has existed since over 5000 years and is widely practised and performed all over the world. However, the semantic meaning of the dance gestures and body postures as well as the intricate steps accompanied by music and recital of poems is only understood fully by the connoisseur. The common masses who watch a concert rarely appreciate or understand the ideas conveyed by the dancer. Can machine learning algorithms aid a novice to understand the semantic intricacies being expertly conveyed by the dancer? In this work, we aim to address this highly challenging problem and propose deep learning based algorithms to identify body postures and hand gestures in order to comprehend the intended meaning of the dance performance. Interestingly, we show with experiments performed using Kinect in constrained laboratory settings and data from Youtube that it is possible to identify body poses and hand gestures of the performer to understand the semantic meaning of the enacted dance piece.
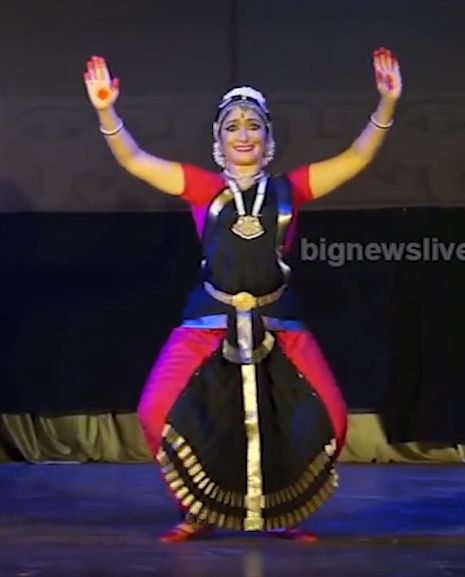
(a)
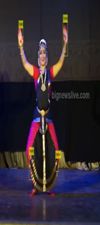
(b)
(a) The hand gestures in a performance of Indian classical dance (ICD) (b) The recognized hand gesture.The recognized hand gesture in the ICD can be fed to our trained model for classifying it followed by semantic interpretation of the same.
Robust pose recognition
The current state-of-the-art pose estimation methods are not flexible enough to model horizontal people, suffers from double counting phenomena (when both left and right legs lie on same image region) and gets confused when objects partially occlude people. Earlier works on pose estimation impose a stickman model on the image of the body and assume that the head lies above the torso. Similarly, shoulder joints are supposed to be higher than the hip joint and legs. However, these assumptions are unrealistic and are violated under typical scenarios shown in this work. As an example, we show how one state-of-the art approach fails to estimate the pose correctly for an image taken from the standard PARSE dataset as shown in figures (a) and (b). The images of Indian classical dance (ICD) and Yoga too have such complex configuration of body postures where current pose estimation methods fail as shown in figures (c) and (d).
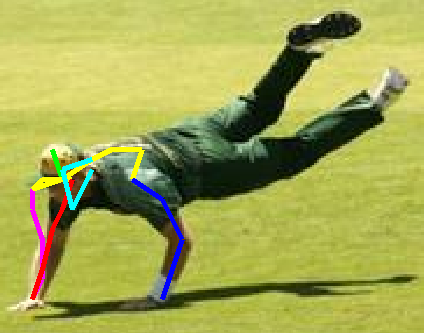
(a)
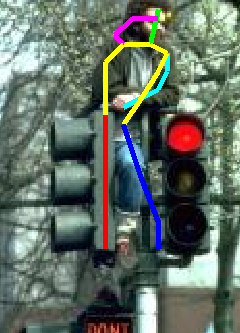
(b)
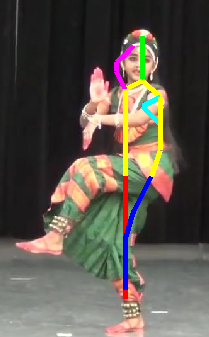
(c)
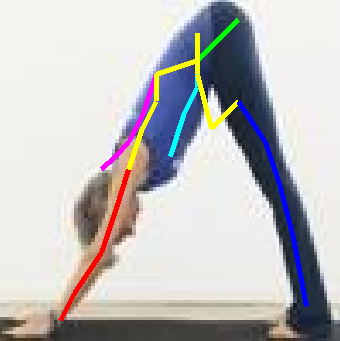
(d)
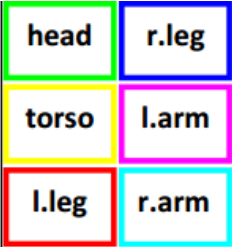
(e)
As shown in the figures above, the state-of-the-art approaches are not robust enough for estimating poses in conditions such as bad illumination, clutter, flowing dress, twisted body commonly found in the images of ICD and Yoga. Hence, the work aims to classify the poses which violate the assumptions made by state-of-the-art approaches.