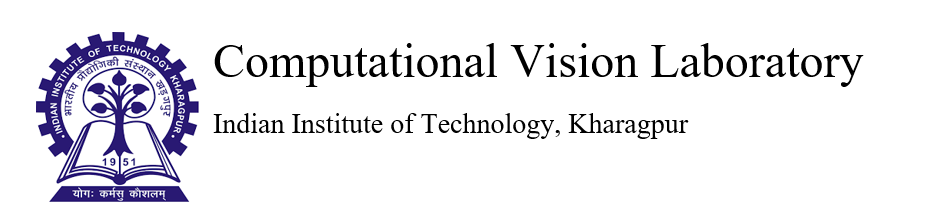
Image de-fencing
Image de-fencing/inpainting is the task of replacing the content of an image or video with some other content which is visually pleasing. Now a days, with the availability of inexpensive smartphones/tablets equipped with cameras has resulted in the average person capturing cherished moments as images/videos and sharing them on the internet. However, at several locations an amateur photographer is frustrated with the captured images. For example, the object of interest to the photographer might be occluded or fenced. Currently available image de-fencing methods in the literature are limited by non-robust fence detection and can handle only static occluded scenes whose video is captured by constrained camera motion. The objective of this research is to resolve the challenges and eventually build an efficient system for the detection and removal offences/occlusions from images/videos. Current objective necessitates the solution of three sub-problems: (a) segmentation of fences/occlusions in the frames of the video, (b) estimation of optical flow between the observations under occlusions, (c) information fusion to fill-in the occluded pixels in the reference image.
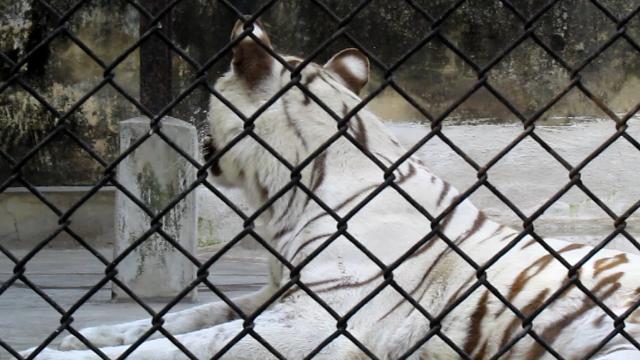
(a) Fenced observation
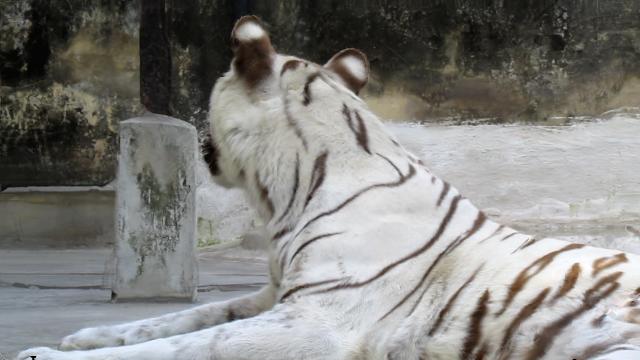
(b) De-fenced image
Semantic segmentation of partially blurred image regions
Detection of blurred and non-blurred regions in a single image without needing to perform blur kernel estimation is a challenging task in computer vision. Semantic segmentation of partially blurred regions in images/videos is another goal of my research. Robust and accurate blurred region segmentation algorithms are useful in many computer vision problems including but not limited to depth of field estimation, image segmentation, object recognition, partial image deblurring, blur magnification, image inpainting and information retrieval etc. In this work, we propose a convolutional neural network (CNN) based algorithm for detection and classification of partially blurred image regions.
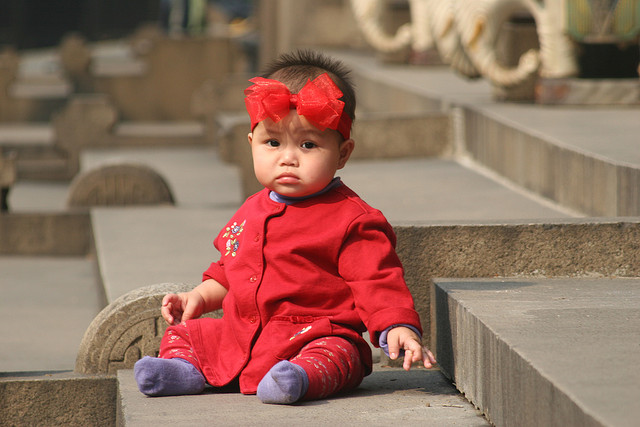
(a) defocussed image
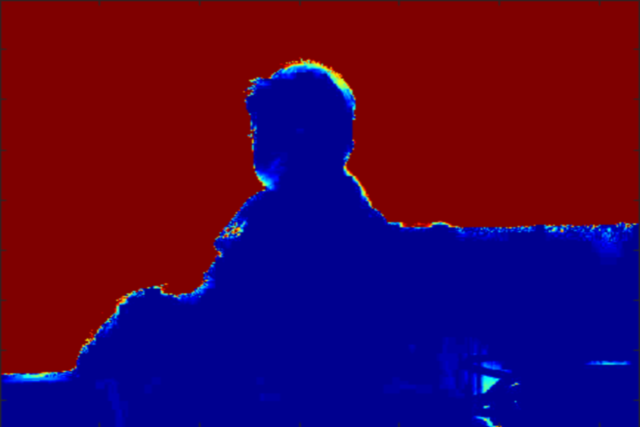
(b) blur map
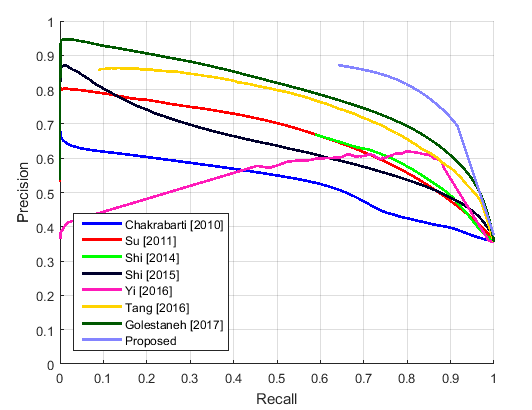
(a)
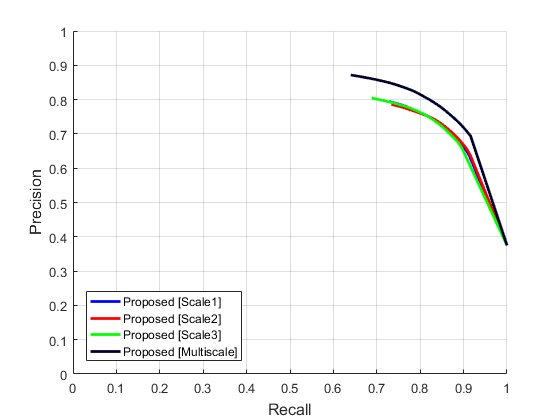
(b)
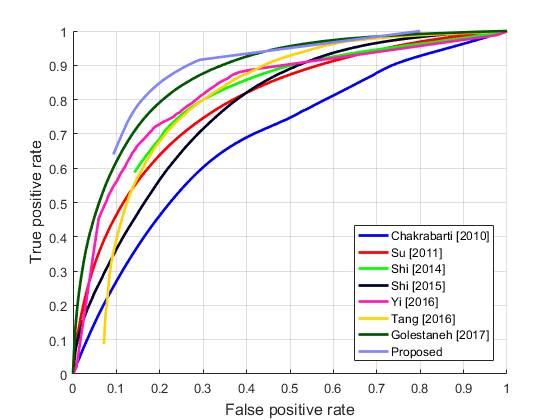
(c)
(a) Precison-recall curves for different algorithms (b) Precison-recall curves between proposed single scale and multiscale superpixel CNN results (c) ROC performance of all methods.